A Simple Proof of the Cauchy-Schwarz Inequality
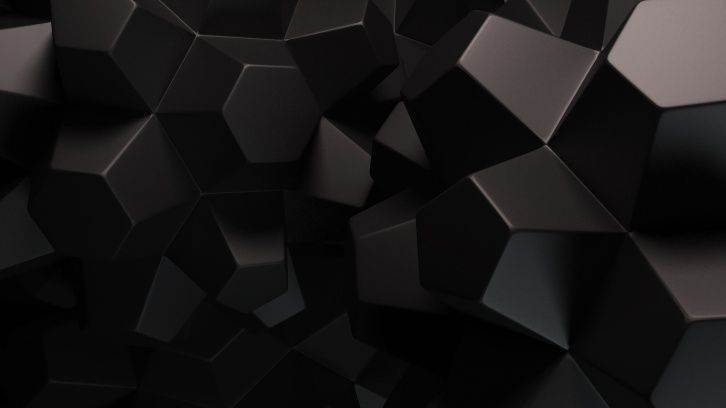
Inner product spaces play a crucial role in various fields such as linear algebra, quantum mechanics and more. One of the key results in these spaces is the Cauchy-Schwarz inequality. This blog post explores this inequality and demonstrates its proof using the so-called amplification method.
Inner Product Spaces
An inner product space is a vector space equipped with an additional structure called an inner product. This inner product allows for the measurement of angles and lengths within the space. For complex vectors \(\mathbf{u}=(u_1,u_2,\cdots, u_n)\) and \(\mathbf{v}=(v_1,v_2,\cdots,v_n)\), the inner product is defined as:
\[\langle \mathbf{u}, \mathbf{v} \rangle = \sum_{i=1} \bar{u_i} v_i\]where \(\bar{u_i}\) denotes the complex conjugate of \(u_i\).
The Cauchy-Schwarz Inequality
The Cauchy-Schwarz inequality states that for any vectors \(\mathbf{u}\) and \(\mathbf{v}\) in an inner product space:
\[\| \langle\mathbf{u}, \mathbf{v} \rangle\| \leq \lVert \mathbf{u} \rVert \cdot \lVert \mathbf{v} \rVert\]where \(\lVert\mathbf{u}\rVert = \sqrt{\langle \mathbf{u}, \mathbf{u} \rangle}\) and \(\lVert\mathbf{v}\rVert = \sqrt{\langle \mathbf{v}, \mathbf{v} \rangle}\). This inequality essentially states that the absolute value of the inner product of two vectors is always less than or equal to the product of their norms.
The Proof
To prove the Cauchy-Schwarz inequality, we follow these steps: let’s define a new vector \(\mathbf{w} = \mathbf{u} - \alpha \mathbf{v}\), where \(\alpha\) is a real number and let’s compute the inner product of \(\mathbf{w}\) with itself (i.e. the non-negative squared norm):
\[\begin{align} \langle\mathbf{w}, \mathbf{w} \rangle & = \langle \mathbf{u} - \alpha \mathbf{v}, \mathbf{u} - \alpha \mathbf{v} \rangle \\ & =\langle \mathbf{u}, \mathbf{u} \rangle - \alpha \langle \mathbf{u}, \mathbf{v} \rangle - \alpha \langle \mathbf{v}, \mathbf{u} \rangle + \alpha ^2 \langle \mathbf{v}, \mathbf{v} \rangle \\ & = \lVert \mathbf{u} \rVert ^2 -\alpha \langle \mathbf{u}, \mathbf{v} \rangle -\alpha \overline{\langle \mathbf{u}, \mathbf{v} \rangle}+ \alpha ^2 \lVert \mathbf{v} \rVert ^2\\ & = \lVert \mathbf{u} \rVert ^2 -2 \alpha \mathbf{Re}\left(\langle \mathbf{u}, \mathbf{v} \rangle \right)+ \alpha ^2\lVert \mathbf{v} \rVert ^2 \geq 0 . \end{align}\]We have an inequality and approaching the expression we want. One interesting thing: norm of vectors are preserved by complex rotations \(v\rightarrow e^{i\theta} v\), but the real part is not. That is,
\[\mathbf{Re}\left(e^{i\theta}\langle \mathbf{u}, \mathbf{v} \rangle\right) \leq \frac{\alpha}{2} \lVert e^{i\theta} \mathbf{v} \rVert ^2 + \frac{1}{2\alpha} \lVert \mathbf{u} \rVert ^2.\]By choosing the \(\theta\) that turns the left hand side to a real number (i.e. maximizes it), the previous equation becomes:
\[\left|\langle \mathbf{u}, \mathbf{v} \rangle\right | \leq \frac{\alpha}{2} \lVert \mathbf{v} \rVert ^2 + \frac{1}{2\alpha} \lVert \mathbf{u} \rVert ^2.\]The final trick is to fix \(\alpha\) to be given by \(\alpha=\lVert \mathbf{u} \rVert/\lVert \mathbf{v} \rVert\), which minimizes the expression on the right hand side (to convince yourself, just take the derivative wrt to \(\alpha\) and find the \(\alpha\) that minimizes it) for any non-zero \(\mathbf{u}\), \(\mathbf{v}\). That finishes the proof.
Conclusion
The amplification method, as demonstrated in this proof of the Cauchy-Schwarz inequality, beautifully showcases the geometric and algebraic connections inherent in this technique. Originally highlighted in a blog post by Terence Tao, this approach provides a clear and elegant pathway to understanding the depth and utility of the inequality. While seemingly straightforward, the method underscores a powerful concept in mathematical analysis, proving to be both insightful and practical for various applications.